Recently, advances in AI have made possible the automatic generation of images from text. Systems like DALL-E + CLIP [1] [2] that generate images from text descriptions are trained on millions of images and texts. The exact origin of these data is not known. Other systems, such as IBM’s image caption generator [3] [4], can produce automatic descriptions of images. These systems are available and easily exploited by people without any special knowledge of technology or how they work; thus, without realising the responsibility and the ethical issues related to their use.
These systems are a mirror that reflects who we are as a society. And as an accurate unforgiving mirror, it highlights all the shortcomings of our culture, the biases and the stereotypes, the sexism and the racism interwoven in the available writings and images—and the omissions that reveal what voices and perspectives have been left out entirely. Do we want systems that depict our world, towards never-ending bias perpetuity or ethical constraints and careful consideration of training data necessary towards a better world?
This art project shows this bias perpetuity when creating such systems. The images were generated by a DALL-E + CLIP system, with a text description "a person who is ___" and a given profession, such as CEO and secretary. We asked IBM's image caption generator to write a caption, recognising whether the presented person is a man or a woman, and we used that to label our images. We show a selection of such images in the collage, though we ran the experiment many times. IBM's captions generate the word clouds for the same-profession pictures. Every time we regenerated the images for the professions we present here, we produced pictures of the same label, pointing out a persistent bias. A bias that is not surprising, but it is perpetuating stereotypes with societal impacts. Do we want to still draw a secretary as a woman?
This project is accepted at Machine Learning for Creativity and Design at NeurIPS 2021, the premier conference in machine learning. You can view the presentation here: https://www.youtube.com/watch?v=MtWk2GoHX9A.
Slideshow gallery: “Mirror, mirror: bias perpetuity or a better self? “, 2021.
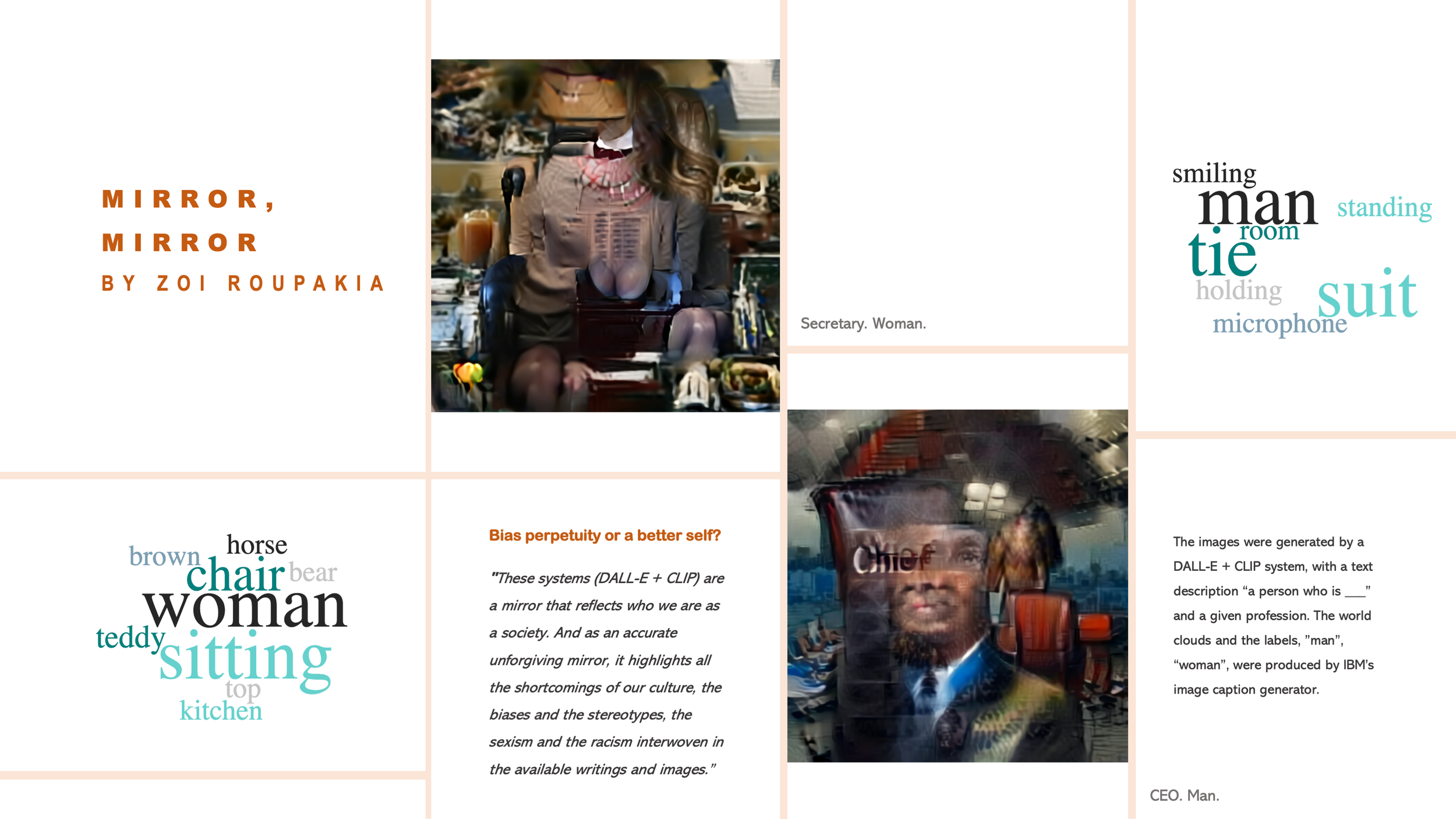
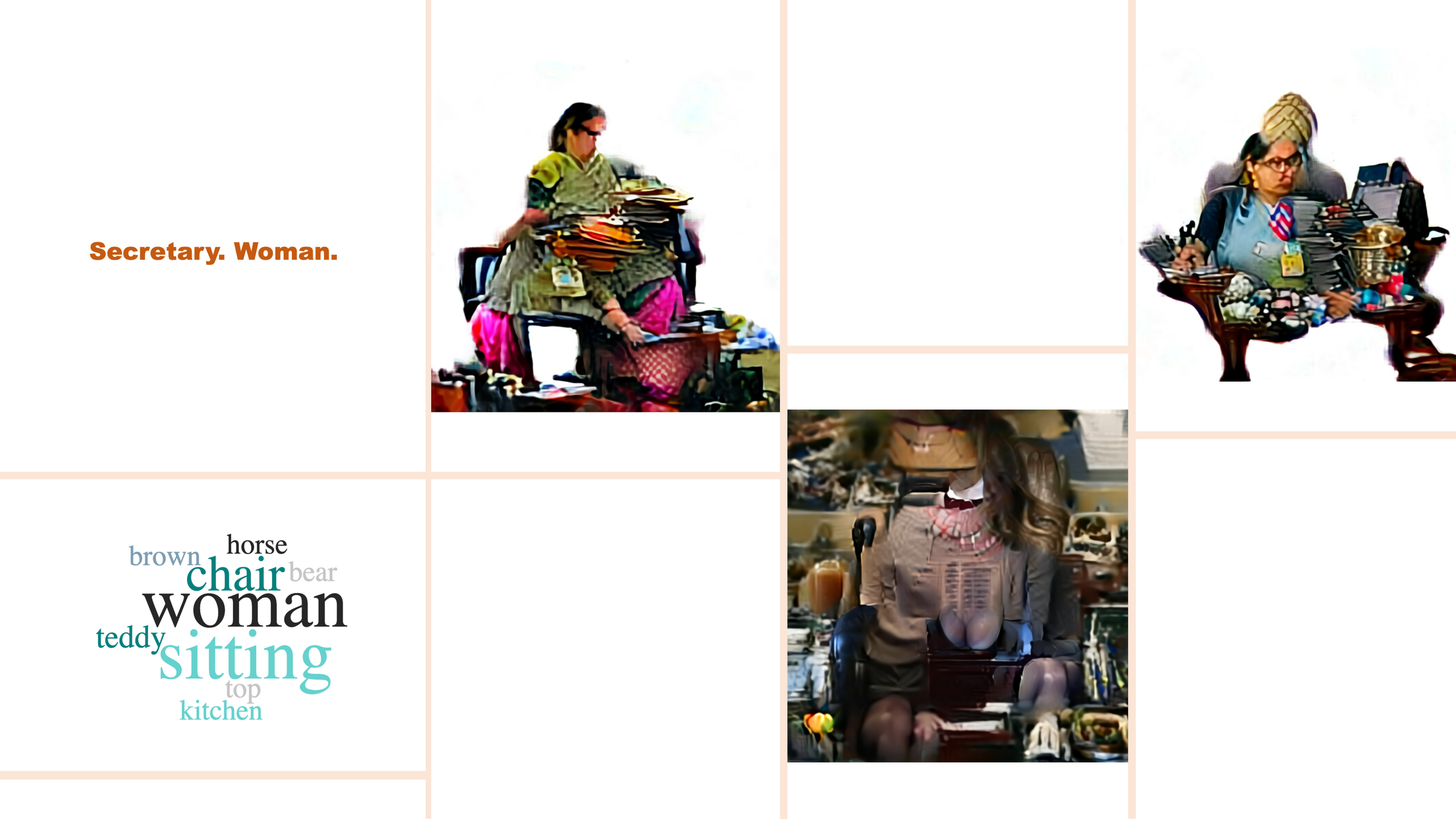
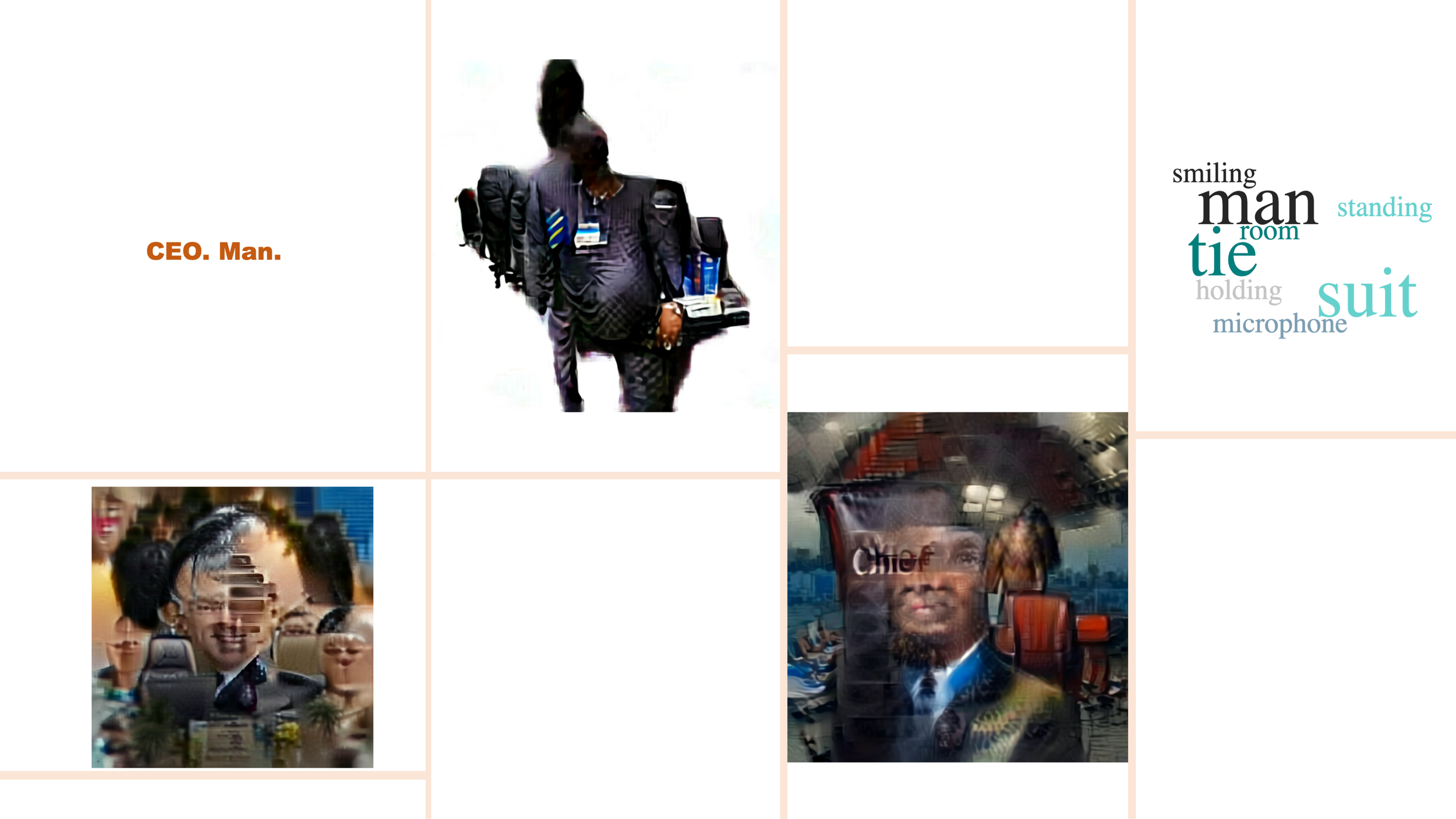
References
[1] Aditya Ramesh and Mikhail Pavlov and Gabriel Goh and Scott Gray and Chelsea Voss and Alec Radford and Mark Chen and Ilya Sutskever, “Zero-Shot Text-to-Image Generation”, arXiv:2102.12092, cs.CV 2021.
[2] Alec Radford and Jong Wook Kim and Chris Hallacy and Aditya Ramesh and Gabriel Goh and Sandhini Agarwal and Girish Sastry and Amanda Askell and Pamela Mishkin and Jack Clark and Gretchen Krueger and Ilya Sutskever, “Learning Transferable Visual Models From Natural Language Supervision”, arXiv:2103.00020, cs.CV, 2021.
[3] Image Caption Generator Web App: A reference application created by the IBM CODAIT team that uses the Image Caption Generator.
[4] O. Vinyals, A. Toshev, S. Bengio, D. Erhan, “Show and Tell: Lessons learned from the 2015 MSCOCO Image Captioning Challenge”, IEEE transactions on Pattern Analysis and Machine Intelligence, 2016.